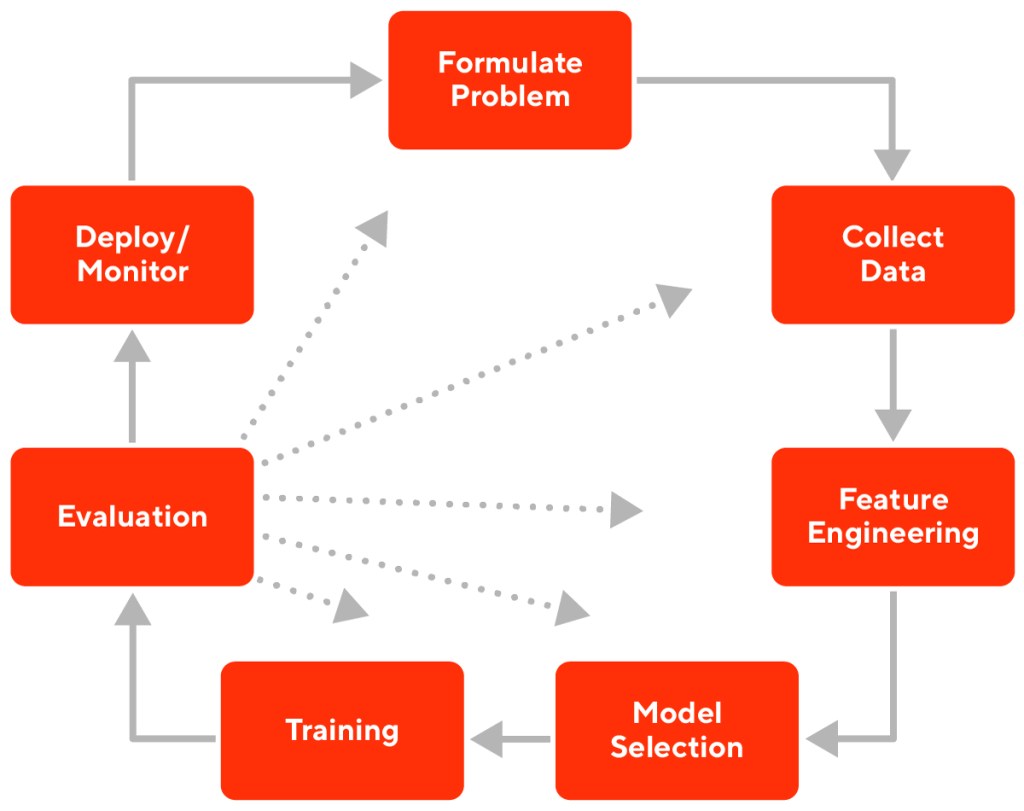
There are fundamental differences between deep-learning and machine learning. The former relies upon unsupervised learning while the latter uses huge datasets and advanced computing tools. Let's take a look at the key differences between the two approaches and how they differ. Understanding the concepts of each is helpful to help you understand the differences. Read this article for more information! We'll also discuss the benefits and drawbacks of each method.
Unsupervised learning
Unsupervised learning, unlike supervised learning that relies upon data tags by humans, uses data sets without tags. Unsupervised learning algorithms can find natural groups or clusters from a set of data. These algorithms, also known as "clustering", can detect correlations between data objects. Another important use of unsupervised learning is anomaly detection, which is used in banking systems to spot fraudulent transactions. Unsupervised learning techniques are becoming more common as people attempt to make computers smarter, better able to complete tasks.
The most obvious difference between unsupervised and supervised methods is in the types of problems for which one approach is better. If there are reference points or ground truth, then supervised learning methods can be used. It's not always easy for people to access clean and clearly labeled data. The algorithms in supervisedlearning are best suited for solving real-world computation issues, while unsupervised methods of learning are best suited in finding interesting patterns within data.
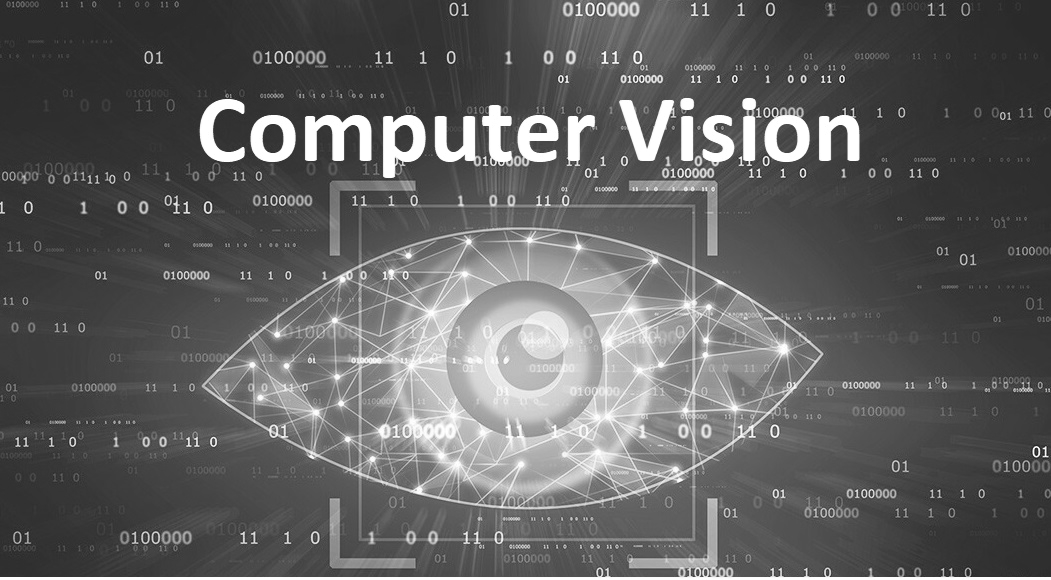
Large data sets
Machine learning can use a variety data types. These data can be divided into four types depending on what task they are being used for. This article will explain the different types of data that are used in machine-learning and how they can be used to help you create a better machine-learning model. This article will also discuss some of the most common methods to extract machine-learning data. Below are the most popular methods to obtain machine learning data.
One of the best ways to get access to large datasets is to look for tutorials online. Kaggle, a community-driven platform, hosts tutorials on hundreds of real-world ML issues. These datasets are usually free and provided by companies, international organisations, and educational institutes like Harvard and Statista. The Registry of Open Data (AWS) is another source of free data. Anyone can post datasets. Once you have access to the data, you can use Amazon data analytics tools to explore it and make it actionable.
Power requirements
The ideal solution for mobile platforms is devices that have AI capabilities. They won't require much power in the near future. These systems require a lot of power, but the details are not known. The cloud providers are not required to disclose their total power consumption for machine-learning systems. Google, Amazon, Microsoft declined comment. AI systems are an exciting new technology but the current power requirements are not sustainable.
Machine learning algorithms require more power as the training datasets increase. A single V100 GPU uses between 250 and 300 watts. A system with 512 GPUs V100 consumes approximately 128,000 watts (128 kilowatts). MegatronLMs were used in one study to train a neural networks. The system consumed 27,648kWh. That's about the same energy as three houses. Machine learning algorithms use less energy thanks to new training techniques. However, many models still require enormous data to train.
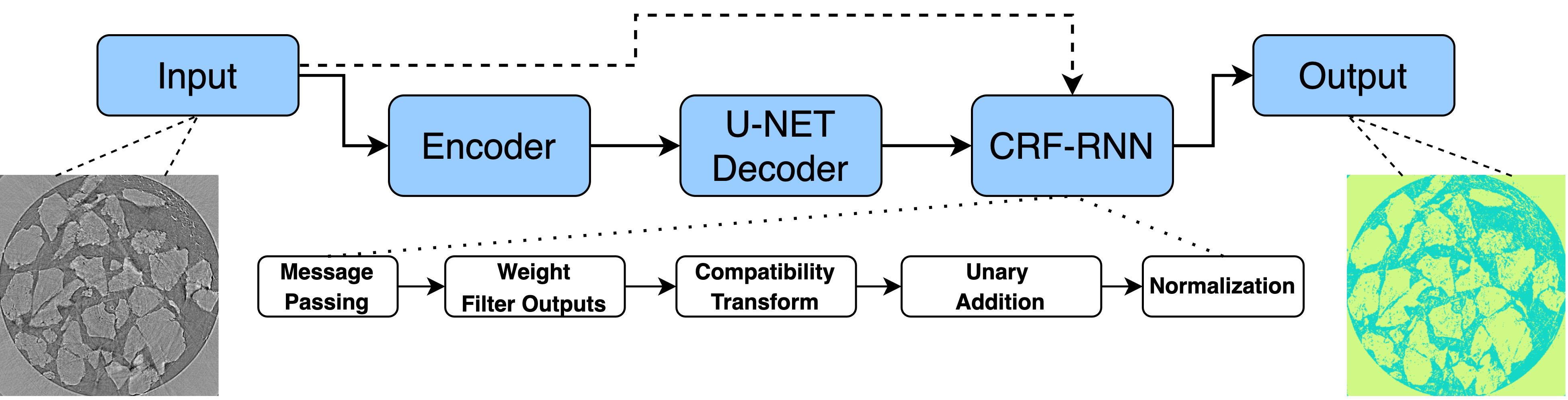
Applications
Machine learning and deeplearning are powerful tools in business intelligence. Semi-autonomous cars employ machine learning algorithms for partially visible objects recognition. And a smart assistant usually combines supervised and unsupervised machine learning models to interpret natural speech and provide context. The use of these techniques is growing rapidly. Continue reading for more information about deep and machine learning.
Social networking platforms like Facebook use machine learning algorithms to automatically classify photos. Facebook creates albums of photos and automatically labels uploaded images. Google Photos applies deep learning to describe every element in a picture. A product recommendation is an example of a Deep Learning app. This technique is used by e-commerce websites to track user behavior and provide product recommendations based upon past purchases. For example, a smart-face lock uses this technology.
FAQ
What is the most recent AI invention?
Deep Learning is the latest AI invention. Deep learning is an artificial intelligent technique that uses neural networking (a type if machine learning) to perform tasks like speech recognition, image recognition and translation as well as natural language processing. Google invented it in 2012.
Google's most recent use of deep learning was to create a program that could write its own code. This was achieved by a neural network called Google Brain, which was trained using large amounts of data obtained from YouTube videos.
This enabled it to learn how programs could be written for itself.
IBM announced in 2015 that they had developed a computer program capable creating music. Music creation is also performed using neural networks. These networks are also known as NN-FM (neural networks to music).
What does AI mean for the workplace?
It will change our work habits. We can automate repetitive tasks, which will free up employees to spend their time on more valuable activities.
It will enhance customer service and allow businesses to offer better products or services.
It will help us predict future trends and potential opportunities.
It will help organizations gain a competitive edge against their competitors.
Companies that fail AI will suffer.
AI: Is it good or evil?
AI is seen both positively and negatively. On the positive side, it allows us to do things faster than ever before. No longer do we need to spend hours programming programs to perform tasks such word processing and spreadsheets. Instead, we just ask our computers to carry out these functions.
Some people worry that AI will eventually replace humans. Many believe that robots could eventually be smarter than their creators. They may even take over jobs.
How does AI work
An artificial neural network consists of many simple processors named neurons. Each neuron takes inputs from other neurons, and then uses mathematical operations to process them.
The layers of neurons are called layers. Each layer serves a different purpose. The first layer receives raw data, such as sounds and images. These data are passed to the next layer. The next layer then processes them further. The final layer then produces an output.
Each neuron is assigned a weighting value. This value gets multiplied by new input and then added to the sum weighted of all previous values. If the result exceeds zero, the neuron will activate. It sends a signal along the line to the next neurons telling them what they should do.
This continues until the network's end, when the final results are achieved.
Statistics
- According to the company's website, more than 800 financial firms use AlphaSense, including some Fortune 500 corporations. (builtin.com)
- In 2019, AI adoption among large companies increased by 47% compared to 2018, according to the latest Artificial IntelligenceIndex report. (marsner.com)
- Additionally, keeping in mind the current crisis, the AI is designed in a manner where it reduces the carbon footprint by 20-40%. (analyticsinsight.net)
- The company's AI team trained an image recognition model to 85 percent accuracy using billions of public Instagram photos tagged with hashtags. (builtin.com)
- A 2021 Pew Research survey revealed that 37 percent of respondents who are more concerned than excited about AI had concerns including job loss, privacy, and AI's potential to “surpass human skills.” (builtin.com)
External Links
How To
How to set up Amazon Echo Dot
Amazon Echo Dot is a small device that connects to your Wi-Fi network and allows you to use voice commands to control smart home devices like lights, thermostats, fans, etc. To begin listening to music, news or sports scores, say "Alexa". You can ask questions, make phone calls, send texts, add calendar events, play video games, read the news and get driving directions. You can also order food from nearby restaurants. It works with any Bluetooth speaker or headphones (sold separately), so you can listen to music throughout your house without wires.
An HDMI cable or wireless adapter can be used to connect your Alexa-enabled TV to your Alexa device. You can use the Echo Dot with multiple TVs by purchasing one wireless adapter. You can also pair multiple Echos at once, so they work together even if they aren't physically near each other.
To set up your Echo Dot, follow these steps:
-
Turn off your Echo Dot.
-
Use the built-in Ethernet port to connect your Echo Dot with your Wi-Fi router. Make sure to turn off the power switch.
-
Open the Alexa app on your phone or tablet.
-
Select Echo Dot in the list.
-
Select Add New.
-
Select Echo Dot from among the options that appear in the drop-down menu.
-
Follow the screen instructions.
-
When prompted, enter the name you want to give to your Echo Dot.
-
Tap Allow Access.
-
Wait until the Echo Dot has successfully connected to your Wi-Fi.
-
For all Echo Dots, repeat this process.
-
Enjoy hands-free convenience